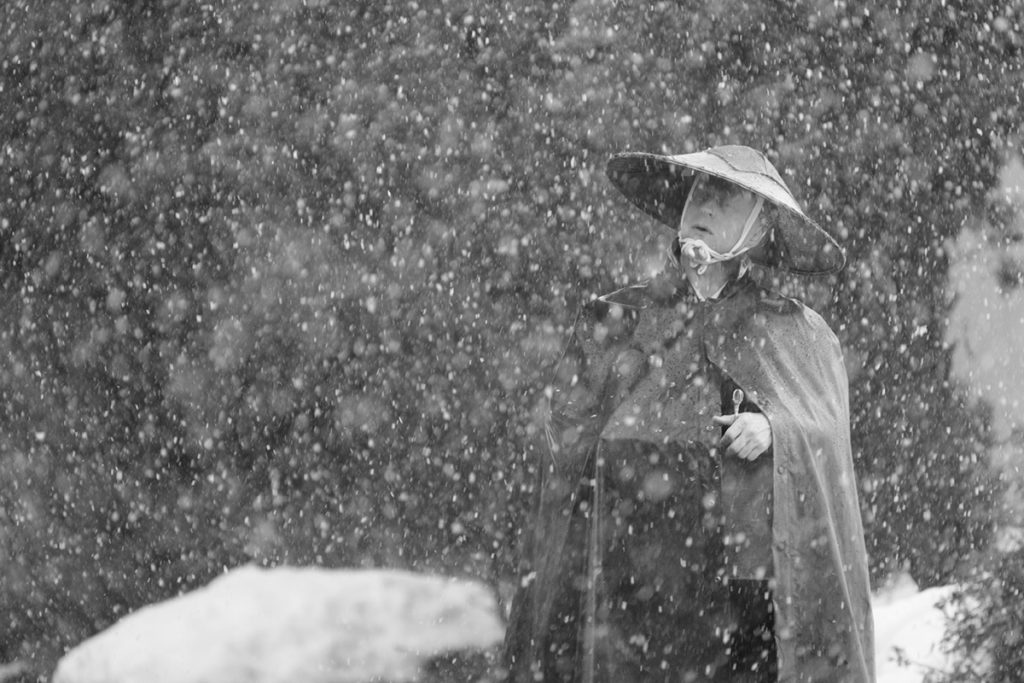
As an Affiliate Fellow working on AI governance at Stanford University’s Institute for Human-Centered AI (HAI), I am well-acquainted with cutting-edge innovations in computing and the entrepreneurial culture of Silicon Valley. As a social scientist trained in the interdisciplinary field of Science & Technology Studies (STS), however, I also know the value of dissenting and under-represented voices in changing the technoscience for the better.
Critical Studies of Artificial Intelligence
I try to bridge this and other knowledge gaps in my research on AI. For instance, I recently guest edited “AI and its Discontents” (March, 2021), a double issue of Interdisciplinary Science Reviews featuring critical analyses of AI from legal scholars, computer scientists, sociologists, historians, and literary experts.
…this special issue brings together critical accounts of AI and its discontents, past and present, in order to capture the significance of this historical moment, expand the horizons of the possible, and catalyze sociotechnical action on behalf of diverse publics and future generations whose autonomy – and humanity – are at stake.”
From the Introduction
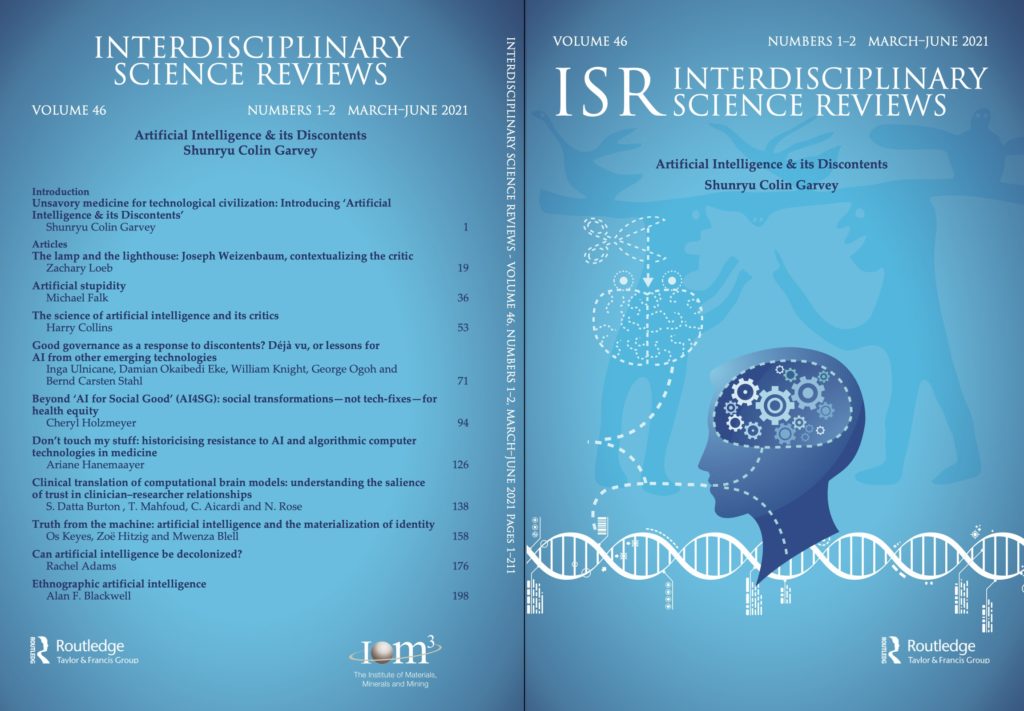
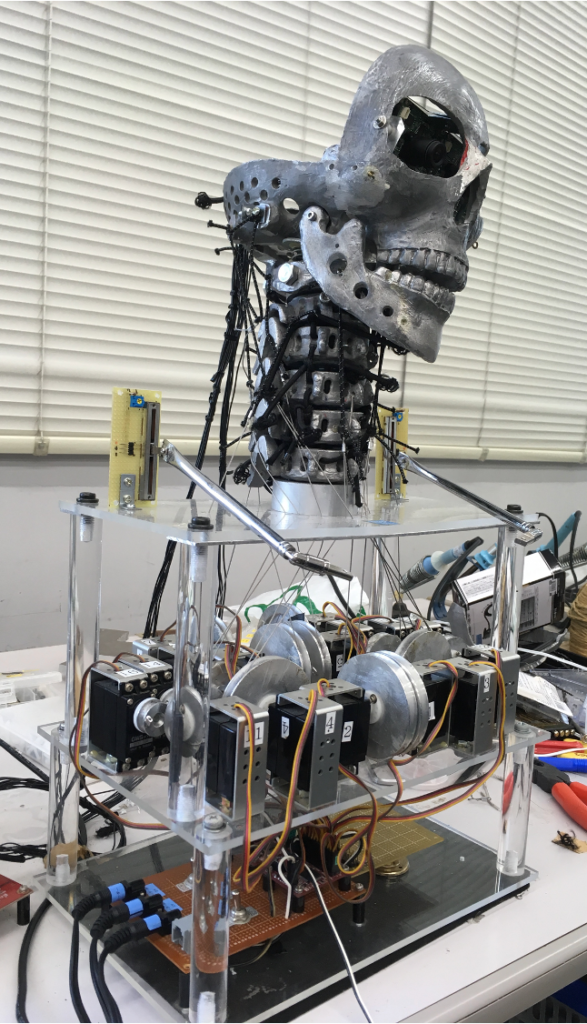
Diagnosing the Terminator Syndrome
I also sometimes combine quantitative and qualitative methods to analyze AI. Calling it the “Terminator Syndrome” Project, Chandler Maskal (a former student of mine at RPI, now at IBM) and I conducted sentiment analysis of news articles about AI. Looking at the New York Times for depth and pulling from the 3000 sources available through NewAPI for breadth, we found most news about AI has a positive valence. Other studies, using far more sophisticated and costly methods, found nearly-identical results.
This was an important finding because it contradicts the claim, often made by AI workers, that their work is harmless, and public concerns about AI arise only because they are being unfairly maligned by a sensationalist media that uses images of killer robots to scare people.
The paper is starting to rack up some citations.
AI History: Three Booms
As an historian of technology, I draw on the past to understand social decision making about risky technologies under the inescapable conditions of complexity, uncertainty, and disagreement. Behind the high-tech imagery and hyped-up dreams of a machine-driven future, AI is a social endeavor, conducted by people, for a variety of reasons, many of them rather mundane.
AI has gone through two “booms” prior to the current buzz of activity in the field. Thus far each has been followed by a “bust” or “AI Winter.” My history of American AI, which explored the sociotechnical circumstances leading to the collapse of the field twice in as many decades, was awarded Best Early Career Paper by the Society for the History of Technology.
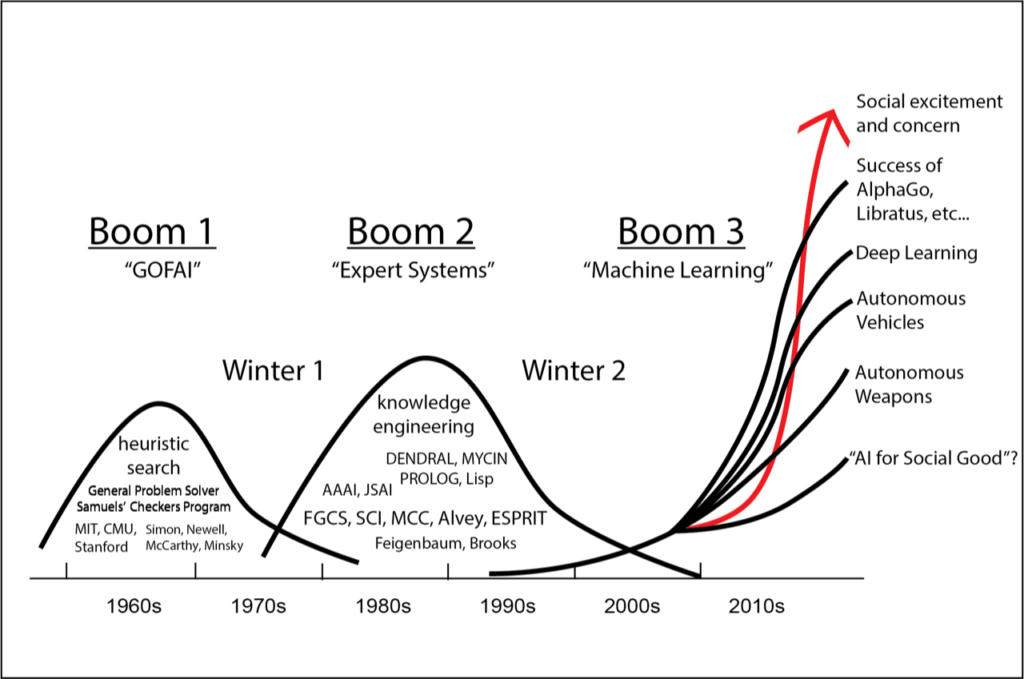
AI Boom 1: Excavating Discontent
Who can help society make sense of the controversial technoscience of AI? The technoscientists whose careers depend on the success of AI? The business people who employ them? The policymakers devoted to the profits promised by the AI-powered “Fourth Industrial Revolution”?
None other than the critics. Little sense can be made of AI without reference to what I call its discontents – those who doubt, question, challenge, reject, reform, and otherwise reprise ‘AI’ as it is practiced, promoted, and (re)produced. With the hope of scaffolding deeper, more nuanced understandings of both the epochal transformations being wrought by AI technologies and the range of responses required, possible, and as-yet unimagined, I dig through the archives in search of little-known and altogether-forgotten figures in the history of AI who still have something to teach us.
One of the most profound discontents I have had the pleasure of finding is Mortimer Taube, a pioneer in information and library sciences, whose vicious attacks on early AI were influential in the first boom, but are little known today.
The science of AI is premised on what Taube called the ‘Man-Machine Identity’: in the words of one AI pioneer the human being is nothing but a ‘meat machine’—albeit a complicated one. Therefore, ‘the simulation of human brains by machines can be interpreted as the simulation of a machine by a machine,’ an operation that is not logically impossible, however improbable, costly, or undesirable. It was at this form of reasoning that Taube leveled his most damning charge: The defense of a research program on the grounds that it is not logically impossible is the hallmark not of Science—but of ‘scientific aberrations’: astrology and phrenology, rather than astronomy or physiology. Just as charlatans claim it is not logically impossible for planetary movements or cranial protuberances to determine human behavior, AI advocates claim it is not logically impossible for machines to simulate cognition.”
AI Boom 2: Global Arms Race
I find the cyclical nature of AI history fascinating. You may have heard about an “AI Arms Race” between the US and China. This is, in fact, the second global AI arms race to take place between the US and an Asian economic powerhouse: Japan was America’s primary rival during the second AI Boom of the 1980s.
This first “AI Arms Race” was integral to the development of AI in Japan, a history I documented pretty extensively here. In the late 1970s, Japan was the world’ second-largest economy and at the top of the semiconductor manufacturing game, having crushed the competition with their “Fourth Generation” VLSI chips. Government bureaucrats began considering the possibility of a “Fifth Generation” of computer hardware that would not only break away from the IBM-dominated, serial von Neumann architecture, but make human-like AI possible. When the “Fifth Generation Computer Systems” (FGCS) Project was finally launched in 1982, however, the West perceived Japan as a threat to its technological supremacy in computing, and responded accordingly.
I think the parallels and difference are instructive for understanding the current competition with China, and presented my thoughts on the subject at the 2020 meeting of the Japanese Society for AI.
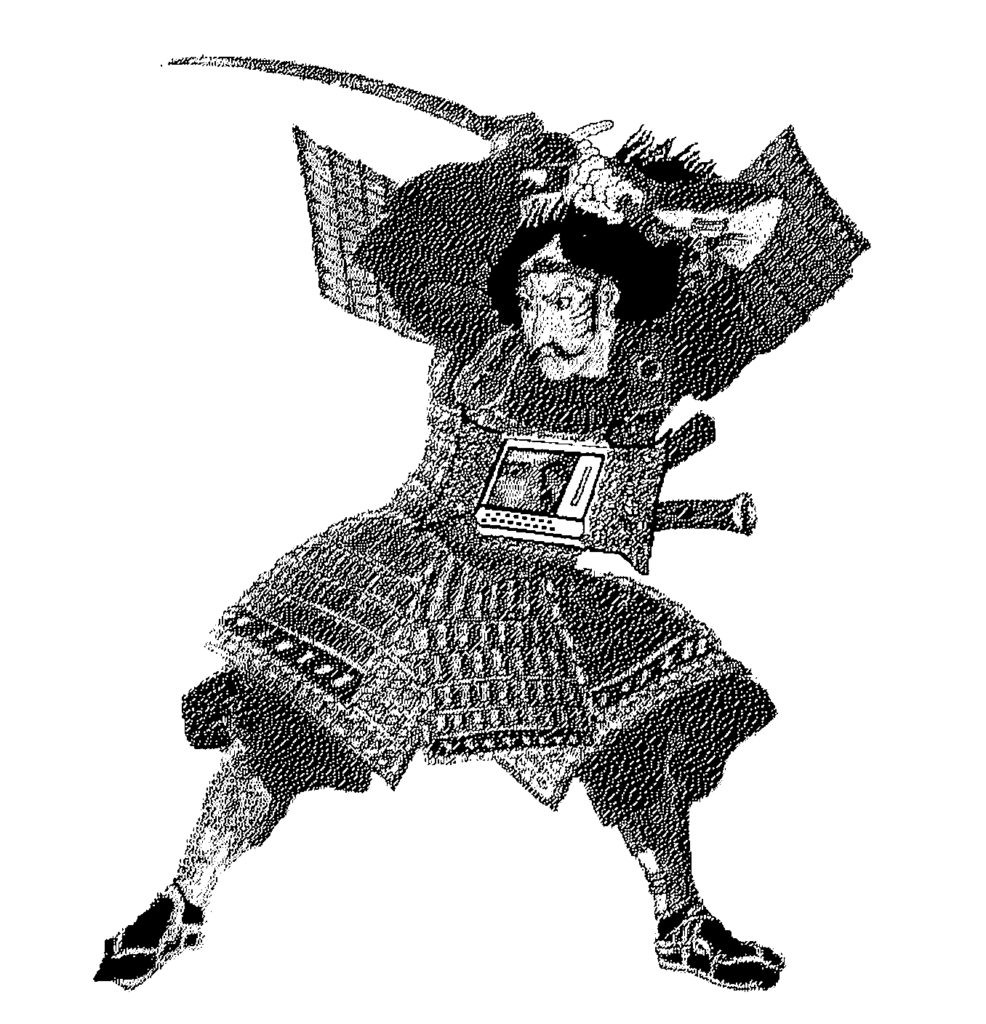
AI Boom 3: Catastrophic Risk Governance
I use history to think about how to shape the future in ways that help humanity, or at least prevent catastrophe. My doctoral dissertation used AI as a case study to explore the bigger question of how societies can more safely, fairly, and wisely govern controversial sociotechnical systems—work that I am currently converting into a book manuscript.
I have presented aspects of this work at the Association for the Advancement of AI, where I introduced a strategic governance framework for improving social decision making about AI, as well as at the inaugural conference on AI, Ethics, and Society, where I presented my typology of catastrophic risks.
My Approach
If you’ve come this far, you are not only brave but probably curious about my approach to AI risk governance. So let’s get into the weeds a little bit, shall we? Here goes:
AI is commonly framed in terms of radical extremes—either utopia or dystopia, heaven or hell.
This ambivalent framing impairs our ability to understand the risks of this emerging technology. Utopians see no risk, while dystopians see only “existential risk,” the danger that AI will somehow make humanity extinct.[i] This absolutism leaves little room to steer AI toward robustly beneficial futures for a majority of humanity: either nothing needs to be done, or nothing can be done.
My research disrupts this polarized framing by articulating seven dimensions of AI risk: military, political, economic, environmental, social, psychophysiological, and existential. I ask of each, Who is creating these risks? Who is being put at risk? Answering these two questions lays the groundwork for my central research question: How can governance be improved to steer AI research and development more safely and more fairly?
I address the first two questions empirically. To understand how AI scientists, developers, entrepreneurs, funders, and users are creating risks, what those risks are, and who is being put at risk, I engaged in participant observation at multiple AI conferences and several laboratories in the USA and Japan, as well as conducted semi-structured interviews with technical experts, social scientists, and workers. In addition, I performed textual analyses on a range of materials, including primary documents from dozens of AI-focused institutions and tech companies; nearly 100 reports on AI from governments and private organizations; video recordings of many AI-themed sessions at conferences (e.g. Davos); numerous user threads in AI-themed online fora; hundreds of tweets from AI scientists and related experts; as well as 2000+ AI-related news items.
Analysis of these materials populated the seven dimensions of what I call the “AI risk horizon” with myriad examples of algorithmic harms, both potential and actual, ranging from the social fallout from malfunctioning facial recognition and automated hiring discrimination to the disastrous economic consequences of massive AI-driven unemployment and the life-threatening development of autonomous weapons systems. I found that in most cases, AI does not introduce entirely new risks per se, but rather amplifies and intensifies the harm caused by existing political, economic, and sociotechnical structures. By scanning the 7D risk horizon, I demonstrate that AI poses serious risks to a majority of humanity not via some mythical “machine takeover,”[ii] but by accelerating the ongoing global ecocide that defines the Anthropocene epoch in which we live.[iii]
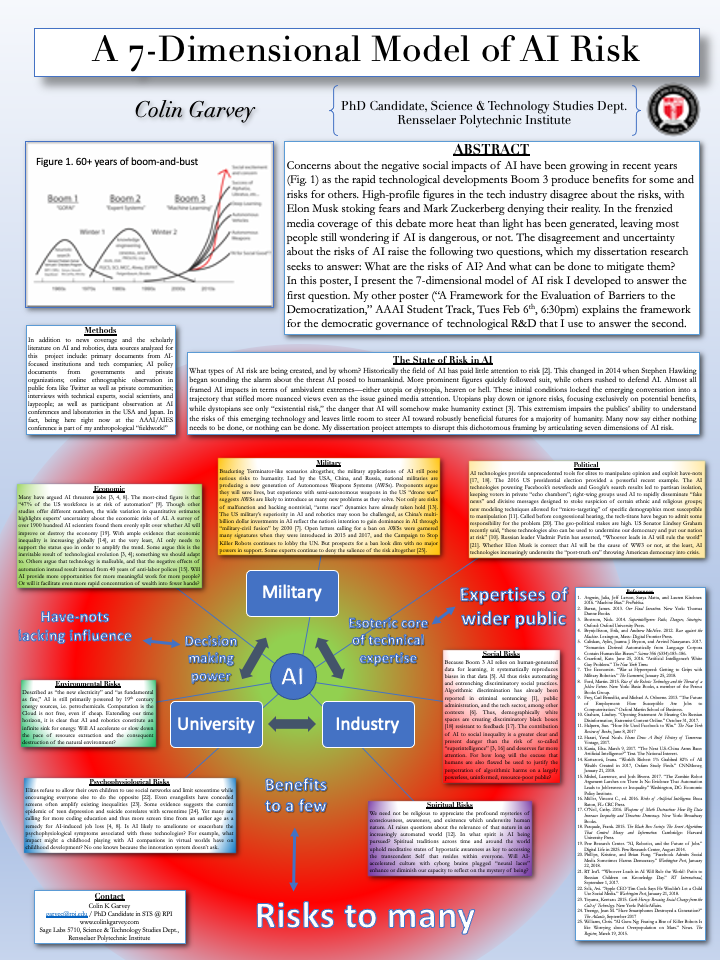
Parsing AI risk along the 7D horizon not only provides a means of tracking the dangers posed by a rapidly developing technology, it also prevents my analysis from reproducing the dystopian alarmism so common in the popular press. There are winners among the losers in each of the seven dimensions of AI risk, from the arms manufacturers who will profit off of killer robots, to the corporate clerks in human resources whose paperwork load is reduced by automated decision making systems. As my findings demonstrate, the balance of AI risk and benefit is not shared equally, but disproportionately distributed along existing lines of power/knowledge.
This assessment of AI risk informs my evaluation of efforts at governance and mitigation. In too many cases, if risks are even acknowledged, the answer to problems created by AI is more and better AI. This technological solutionism affects most of the mitigation strategies I have observed in my fieldwork; consequently, they tend to ignore the economic, social, and political contexts in which the decision making processes leading to the production of AI risk take place. Because they fail to address these issues, I argue that purely technical approaches to risk mitigation are insufficient; attention should also be paid to the politics of technology. I therefore adopt a sociotechnical approach to AI governance in order to better clarify how risks emerge from the political structure of the decision making processes in R&D, and how that might change.
What changes to the governance of AI might help mitigate its many risks? Here I employ literature from Science & Technology Studies (STS) to identify barriers to improved governance of AI and propose strategies for overcoming them. Specifically, I utilize Woodhouse’s framework for democratic decision making through intelligent trial and error (the “ITE Framework”),[iv] which provides a design-based approach to the governance of technological R&D. It originated from case studies of risky 20th century technologies, such as civilian nuclear power, recombinant DNA, and petrochemistry, all of which could have resulted in catastrophic failure and massive losses of life, but did not. Woodhouse and collaborators’ empirical analyses of these cases produced a set of strategies for averting catastrophe.[v] The ITE framework emerged from the synthesis of these strategies with insights from critical scholars of science and technology[vi] and democratic political theorists.[vii] It consists of twenty variables grouped into five strategies for the democratization of technological governance, which I take up in the body chapters of the dissertation. Using the variables, I evaluate AI R&D at multiple scales, from individual projects to initiatives led by multinational corporations, and propose possible policies for democratic change.
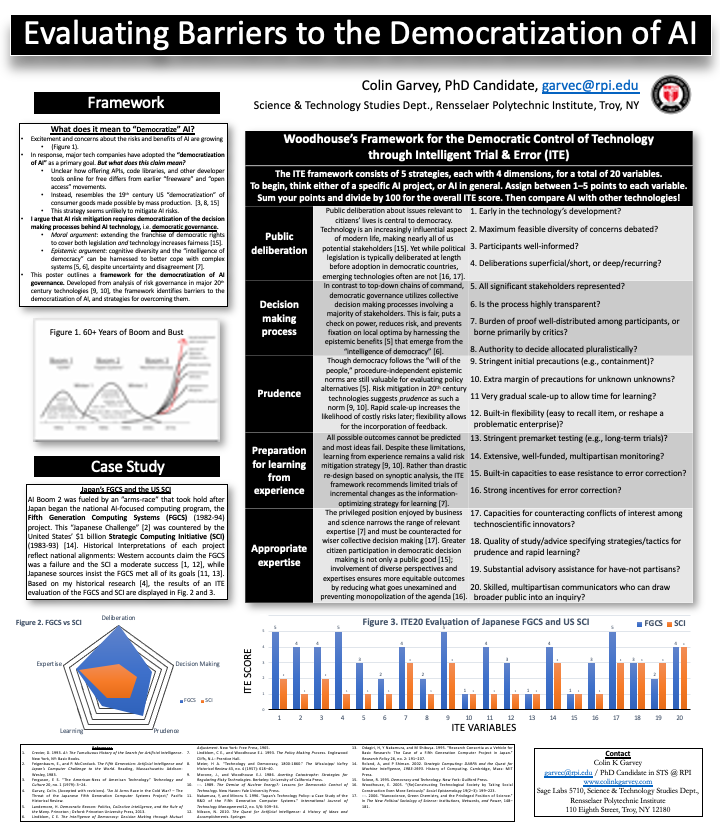
The main takeaway of my research is that more democratic governance of AI offers the best chance of mitigating risk across all seven dimensions of the horizon.
Codes of ethics and other aspirational documents offer excellent models for behavior, but history has shown that democracy is the best check on power, and this is no less true in the 21st century. Moving from description towards prescription by arguing for thoughtful partisanship on behalf of more democracy in technology, my work contributes to the reconstructivist tradition within my field of Science and Technology Studies, i.e. the lineage of thinkers who, having agreed that science and technology are socially constructed, ask how they might be reconstructed more safely, fairly, and wisely.[viii]
[i] James Barrat, Our Final Invention: Artificial Intelligence and the End of the Human Era (New York: Thomas Dunne Books, 2013); Nick Bostrom, Superintelligence: Paths, Dangers, Strategies (Oxford: Oxford University Press, 2014); John Bohannon, “Fears of an AI Pioneer,” Science 349, no. 6245 (July 17, 2015): 252–252, https://doi.org/10.1126/science.349.6245.252; Jim Davies, “Program Good Ethics into Artificial Intelligence,” Nature538 (October 20, 2016): 291, https://doi.org/10.1038/538291a; Vincent C. Müller, ed., Risks of Artificial Intelligence (Boca Raton, FL: CRC Press, 2016); Marco della Cava, “Elon Musk Says AI Could Doom Human Civilization. Zuckerberg Disagrees. Who’s Right?,” USA TODAY, January 2, 2018, https://www.usatoday.com/story/tech/news/2018/01/02/artificial-intelligence-end-world-overblown-fears/985813001/; Ceri Parker, “AI Will Save Us, Not Destroy Us According to Google’s CEO,” World Economic Forum, January 24, 2018, https://www.weforum.org/agenda/2018/01/google-ceo-ai-will-be-bigger-than-electricity-or-fire/; George Dvorsky, “How We Can Prepare for Catastrophically Dangerous AI—and Why We Can’t Wait,” Gizmodo, December 5, 2018, https://gizmodo.com/how-we-can-prepare-now-for-catastrophically-dangerous-a-1830388719.
[ii] Karel Čapek, R.U.R. (Rossum’s Universal Robots), trans. Paul Selver and Nigel Playfair, Dover Thrift Editions (Mineola, N.Y: Dover Publications, 2001); Michael Denton et al., Are We Spiritual Machines?: Ray Kurzweil vs the Critics of Strong A.I., ed. Jay W. Richards, 1st ed (Seattle, WA: Discovery Institute Press, 2002); Robert M. Geraci, Apocalyptic AI: Visions of Heaven in Robotics, Artificial Intelligence, and Virtual Reality (New York: Oxford University Press, 2010); Yuval Noah Harari, Homo Deus: A Brief History of Tomorrow (Vintage, 2017).
[iii] Rodolfo Dirzo et al., “Defaunation in the Anthropocene,” Science 345, no. 6195 (July 25, 2014): 401–6, https://doi.org/10.1126/science.1251817; Simon L. Lewis and Mark A. Maslin, “Defining the Anthropocene,” Nature 519, no. 7542 (March 12, 2015): 171–80, https://doi.org/10.1038/nature14258; Gerardo Ceballos, Paul R. Ehrlich, and Rodolfo Dirzo, “Biological Annihilation via the Ongoing Sixth Mass Extinction Signaled by Vertebrate Population Losses and Declines,” Proceedings of the National Academy of Sciences, July 10, 2017, 6089–6096, https://doi.org/10.1073/pnas.1704949114.
[iv] Edward J. Woodhouse, “Sophisticated Trial and Error in Decision Making About Risk,” in Technology and Politics, ed. Michael E. Kraft and Norman J. Vig (Durham: Duke University Press, 1988), 208–23; Edward Woodhouse and David Collingridge, “Incrementalism, Intelligent Trial-and-Error, and the Future of Political Decision Theory,” in An Heretical Heir of the Enlightenment: Politics, Policy, and Science in the Work of Charles E. Lindblom, 1993, 131–54, http://spp.oxfordjournals.org/content/34/2/139.short.
[v] Joseph G. Morone and Edward J. Woodhouse, Averting Catastrophe: Strategies for Regulating Risky Technologies (Berkeley: University of California Press, 1986); The Demise of Nuclear Energy? Lessons for Democratic Control of Technology (New Haven: Yale University Press, 1989); E. J. Woodhouse and Patrick W. Hamlett, “Decision Making About Biotechnology: The Costs of Learning from Error,” in The Social Response to Environmental Risk (Springer, 1992), 131–150, http://link.springer.com/chapter/10.1007/978-94-011-2954-1_6; Edward Woodhouse and Daniel Sarewitz, “Science Policies for Reducing Societal Inequities,” Science and Public Policy 34, no. 2 (2007): 139–150.
[vi] Lewis Mumford, “Authoritarian and Democratic Technics,” Technology and Culture 5, no. 1 (1964): 1–8; The Myth of the Machine: The Pentagon of Power (New York: Harcourt, Brace, 1970); Hannah Arendt, The Human Condition, 2nd ed (Chicago: University of Chicago Press, 1998); Langdon Winner, Autonomous Technology: Technics-out-of-Control as a Theme in Political Thought, Seventh printing (Cambridge, Massachusetts: MIT Press, 1989); Donna Haraway, “Situated Knowledges: The Science Question in Feminism and the Privilege of Partial Perspective,” Feminist Studies, 1988, 575–599; Sandra G. Harding, Is Science Multicultural?: Postcolonialisms, Feminisms, and Epistemologies (Bloomington, IN: Indiana University Press, 1998); Andrew Feenberg, Technosystem: The Social Life of Reason (Cambridge, Massachusetts: Harvard University Press, 2017).
[vii] Robert Alan Dahl, Dilemmas of Pluralist Democracy: Autonomy vs. Control, Yale Studies in Political Science 31 (New Haven: Yale Univ. Press, 1982); Robert A Dahl, On Democracy (New Haven: Yale University Press, 1998); Charles E. Lindblom, “The Science of ‘Muddling Through,’” Public Administration Review 19, no. 2 (1959): 79, https://doi.org/10.2307/973677; Charles E. Lindblom, The Intelligence of Democracy: Decision Making through Mutual Adjustment (New York: Free Press, 1965); Charles E. Lindblom, “Still Muddling, Not yet Through,” Public Administration Review 39, no. 6 (1979): 517–526; Charles Edward Lindblom, Inquiry and Change: The Troubled Attempt to Understand and Shape Society (New Haven (Conn.): Yale University Press, 1990).
[viii] Edward Woodhouse et al., “Science Studies and Activism: Possibilities and Problems for Reconstructivist Agendas,” Social Studies of Science32, no. 2 (April 1, 2002): 297–319, https://doi.org/10.1177/0306312702032002004; Edward Woodhouse, “(Re)Constructing Technological Society by Taking Social Construction Even More Seriously,” Social Epistemology 19, no. 2–3 (January 2005): 199–223, https://doi.org/10.1080/02691720500145365; David J. Hess, Alternative Pathways in Science and Industry: Activism, Innovation, and the Environment in an Era of Globalization, Urban and Industrial Environments (Cambridge, Mass: MIT Press, 2007); Taylor Dotson, “Technological Determinism and Permissionless Innovation as Technocratic Governing Mentalities: Psychocultural Barriers to the Democratization of Technology,” Engaging Science, Technology, and Society 1, no. 0 (December 29, 2015): 98–120; Donna Jeanne Haraway, Staying with the Trouble: Making Kin in the Chthulucene, Experimental Futures: Technological Lives, Scientific Arts, Anthropological Voices (Durham: Duke University Press, 2016); John Urry, What Is the Future? (Cambridge, UK ; Malden, MA: Polity Press, 2016); Steve Breyman et al., “STS and Social Movements: Pasts and Futures,” in Handbook of Science and Technology Studies, ed. Ulrike Felt et al., 4th ed. (Cambridge, MA: MIT Press, 2017), 289–318; Sigrid Schmalzer, Daniel S. Chard, and Alyssa Botelho, eds., Science for the People: Documents from America’s Movement of Radical Scientists (Amherst: University of Massachusetts Press, 2018).
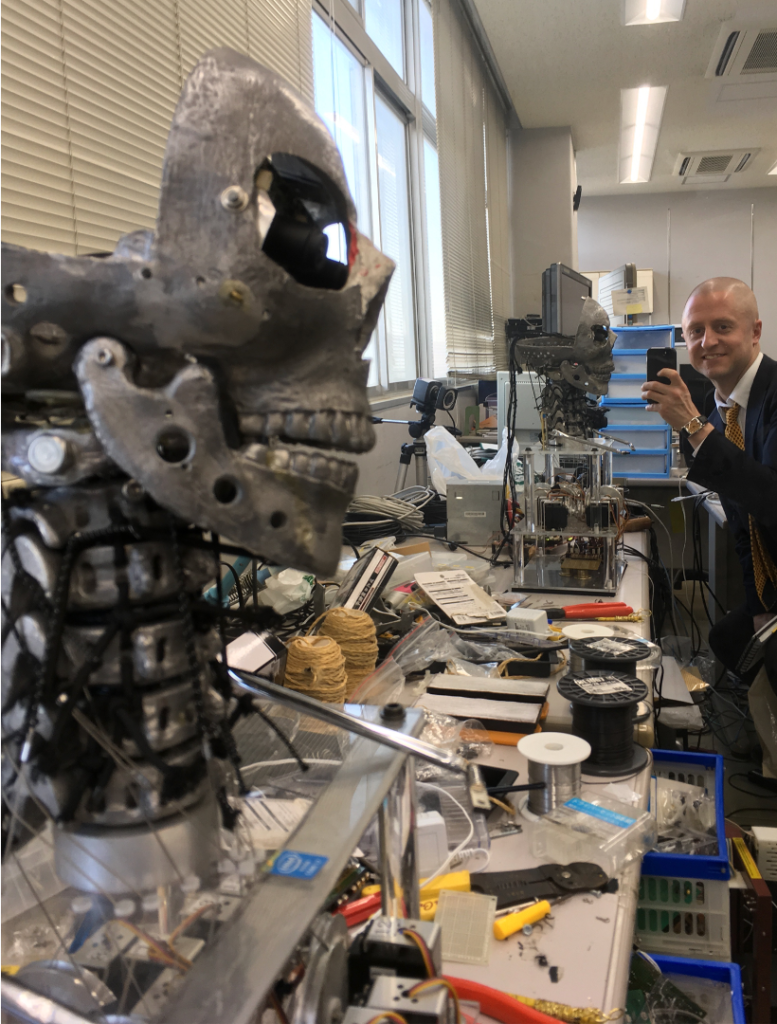